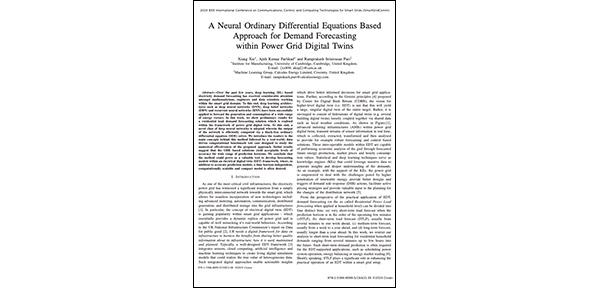
Submitted by Angela Walters on Wed, 23/10/2019 - 16:29
Abstract
Over the past few years, deep learning (DL) based electricity demand forecasting has received considerable attention amongst mathematicians, engineers and data scientists working within the smart grid domain. To this end, deep learning architectures such as deep neural networks (DNN), deep belief networks (DBN) and recurrent neural networks (RNN) have been successfully applied to forecast the generation and consumption of a wide range of energy vectors. In this work, we show preliminary results for a residential load demand forecasting solution which is realized within the framework of power grid digital twin. To this end, a novel class of deep neural networks is adopted wherein the output of the network is efficiently computed via a black-box ordinary differential equation (ODE) solver. We introduce the readers to the main concepts behind this method followed by a real-world, data driven computational benchmark test case designed to study the numerical effectiveness of the proposed approach. Initial results suggest that the ODE based solutions yield acceptable levels of accuracy for wide range of prediction horizons. We conclude that the method could prove as a valuable tool to develop forecasting models within an electrical digital twin (EDT) framework, where, in addition to accurate prediction models, a time horizon independent, computationally scalable and compact model is often desired.
People
Theme
Citation
Xie, X., Parlikad, A.K. and Puri, R.S., 2019, October. A Neural Ordinary Differential Equations Based Approach for Demand Forecasting within Power Grid Digital Twins. In 2019 IEEE International Conference on Communications, Control, and Computing Technologies for Smart Grids (SmartGridComm) (pp. 1-6). IEEE.