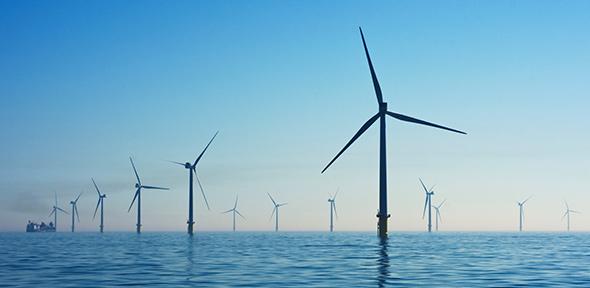
Submitted by Angela Walters on Mon, 15/02/2021 - 10:46
Following publication of his collaborative landmark research synthesising computational mathematics and data science in engineering, Professor Mark Girolami, Sir Kirby Laing Professor of Civil Engineering, Royal Academy of Engineering Research Chair at the University of Cambridge, Programme Director for Data-Centric Engineering at The Alan Turing Institute and Academic lead at both CDBB and the Centre for Smart Infrastructure and Construction (CSIC), welcomes the many potential diverse applications in an increasingly data abundant world.
It all starts with the Finite Element Method (FEM) – a well-established mathematical method used as a computational predictive tool for more than 70 years – which emerged from a need to solve complex problems in structural engineering. Similarly our development of a data-centric FEM, the Statistical FEM (statFEM), that blends mathematical models with data driven models and provides the theoretical foundations by which Digital Twins can be realised, came from a need to solve engineering problems arising from waves that develop and grow under the surface of the oceans, the dreaded solitons.
Two years ago I was heading up a research team at the Turing which was collaborating with the Centre for Offshore Foundation Systems at the University of Western Australia in Perth. We were working with Shell engineers to explore ways to better control the safety, maintenance and management of offshore structures, such as wind turbines for power generation and floating liquified natural gas facilities in the Indian Ocean about 300 miles off the coast of Western Australia.
The ocean conditions there are such that very large amplitude internal waves occur on Australia’s North West Shelf. Known as solitons, these underwater waves contain huge amounts of energy and can create underwater conditions akin to storms. The power of a soliton should not be underestimated; if one of these waves hits a wind turbine or a ship the effects can be incredibly damaging – even fatal.
The team was asked to help predict the chances of one of these solitons coming close to offshore assets. This information would help engineers better manage the safety of their assets and mitigate hazardous conditions. This collaboration resulted in a tool, basically an app running on an iPad to help engineers predict conditions, but it also led to a realisation beyond the project in hand.
Two aspects were striking. The engineers were using many different types of sensors and measurement systems to obtain information about ocean and weather conditions as well as the structural health of their assets – and this process created a lot of data. However, when the wind turbines and gas processing platforms are designed and tested, computational mathematical models describing the structures are used to simulate how they will perform under, in this case, certain wind loadings and other influences. Such mathematical descriptions provide the way to solve these engineering problems and answer a number of crucial questions. And in order to solve the mathematical equations that describe these engineering problems the engineering community uses the FEM to use computer simulations to play out any number of ‘what if’ scenarios.
We were missing an opportunity here. What if we were able to take the computer realisation of the structures and the data that comes from the sensors on those assets and the surrounding environment and integrate both in a formal way to create a much stronger tool?
Why would that be worth doing? The mathematical descriptions used in engineering tend to be idealised and therefore in many cases mis-specified, unable to capture all details of what, for example, the actual wind turbine in the hostile ocean is really like. But the data does tell us that. If we could synthesise the data with the mis-specified mathematical descriptions, we could correct for that idealisation and potentially improve the accuracy of our predictions for the performance of the asset of interest. Of course, it can also work in the opposite direction enabling the mathematical model to inform regions where there is little informative data.
We purposely looked at how we could do this in an abstract way to build a very general theoretical framework applicable to a wide range of settings beyond structural assets. It took almost two years to develop the statFEM and it was a collaborative endeavour greatly helped by working with FEM expert Dr Fehmi Cirak and his research group at Cambridge. When the method was published in an engineering journal it caught the eye of the research team back in Perth, who got in touch and asked if the approach could be applied to the solitons to gain a better understanding of how they evolve.
My team took up the challenge and demonstrated the method in this particular context – the findings are now published in the Proceedings of the National Academy of Sciences (PNAS). The beauty of the statFEM is its generality and versatility; engineers can use the method for realising Digital Twins while scientists that study, for example, electromagnetism or the atmospheric conditions – who commonly use the FEM to simulate their systems – are also gathering data. The potential across all of the sciences goes far beyond our original engineering interests in Western Australia.
Essentially this method lays down the theoretical foundations for the realisation of Digital Twins – the realistic digital representation of assets, processes and systems which are ‘coupled’ via data. Up until now there was no real theory as to how to couple the physical with the virtual world. The statFEM provides that gateway and alongside continuing theoretical developments, we are already demonstrating the possibilities by building a prototype Digital Twin of a railway bridge in Staffordshire on Network Rail’s West Coast Main Line that forms part of an ongoing CDBB and Construction Innovation Hub collaborative project led by the Centre for Smart Infrastructure and Construction (CSIC), along with the Laing O’Rourke Centre for Construction Engineering and Technology, The Alan Turing Institute, and Microsoft.
The bridge is comprehensively instrumented with fibre optic sensors that provide continuous and rich data sets, which will be assessed and modelled by structural engineers at Cambridge. Combining structural and data-driven engineering brings new opportunities to smart infrastructure and with the added tool of the statFEM, we are now building a full Digital Twin of this bridge. Using statFEM to represent the digital replica and synthesising this with the data coming from the sensors on the bridge, the Digital Twin will sit on Microsoft cloud infrastructure to enable asset managers at Network Rail to better understand the structural behaviour of the bridge – and make better decisions to monitor and manage the asset. When completed the Staffordshire bridge will be the first remotely monitored structural asset on the UK rail network.
This research is an excellent example with respect to the fusion of industry and academia. As a Construction Innovation Hub digital twin demonstrator project, the team developed a tangible example of how whole-life value of assets can be measured, tracked and managed in partnership with Network Rail. We hope that work will encourage other asset owners to develop their own whole-life value based on the new digital tools based on statistical FEM. This is an exciting landmark – the ability to combine data and models in a truly data-centric engineering manner brings the opportunity of truly transformative change. And the impact is not confined to one area but will be widespread and far reaching.
Related project: Research Profile - Staffordshire Bridges – long-term performance monitoring using fibre optic sensors
Contact: Professor Mark Girolami