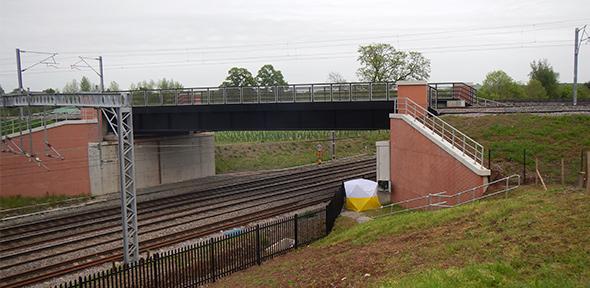
Submitted by Anonymous on Thu, 22/09/2022 - 15:36
A major rail upgrade programme to remove a bottleneck on the West Coast Main Line saw collaboration between Atkins, Laing O’Rourke, Volker Rail and Network Rail. The Stafford Area Improvement Programme included the construction of 10 new bridges designed by Atkins using Building Information Modelling (BIM) from the outset and off-site construction techniques wherever possible. The Staffordshire Alliance team handed over the £250m upgrade in 2015, including 10 kilometres of new line, under budget and more than a year ahead of schedule [CSIC 2016]. As part of the project, CSIC instrumented two of the bridges to create the UK’s first smart railway bridges. CDBB funding enabled researchers to build on this work to create live ‘digital twins’ to reveal the true capacity of the UK’s rail bridges.
Researchers at the Centre for Smart Infrastructure and Construction (CSIC) and the Laing O’Rourke Centre for Construction Engineering and Technology (LORC) were involved in the Network Rail project from the start. They instrumented two of the new bridges with fibre optic sensors to better understand their structural behaviour from the beginning of their service life.
The monitoring systems on the bridges generate vast amounts of data. CSIC/LORC researchers developed a digital twin platform to analyse these rich data sets. The project demonstrates the use of real-time data analytics integrated with digital twins to provide useful information to support engineers and asset managers to schedule safer and proactive maintenance programmes and optimise future designs. Working with Network Rail, the project yielded insightful information about the real-time load and capacity of the bridges with implications for increasing utilisation of the UK rail infrastructure.
Big picture
Bridges are critical structures that connect communities and form a significant part of our national infrastructure network and associated services. Many bridges are ageing and nearing the end of their designed service life while carrying significantly more and heavier vehicles than originally expected. In addition, bridges are exposed to harsher environmental conditions due to the effects of climate change which can lead to further deterioration. Maintenance and repairs can be costly: according to an annual survey by the RAC Foundation and ADEPT (the Association of Directors of Environment, Planning and Transport), the one-off cost of the total maintenance backlog for 71,505 council-managed road bridges in Britain is £5.55bn [RAC 2020].
The current practice of asset management and maintenance of bridges principally relies on information obtained through periodic visual inspections as a basis for establishing repair and maintenance programmes [CDBB].
The case for change
Bridges are complex structures with hard-to-see details and are exposed to changing temperatures, moisture and dynamic loading, making them difficult to inspect. Manual bridge inspection is a subjective, laborious, incomplete and costly procedure. In addition, inspections near busy roads or railways, or at height or over water, bring additional risk and require protective measures and equipment such as mobile elevating work platforms. Collecting and analysing data continuously from a bridge monitoring system provides greater levels of detail and information without the health and safety risks associated with on-site visual inspections.
The project
Researchers collaborated with Network Rail to deliver the UK's first truly smart bridges. The structural configurations of the two monitored bridges are common types that exist on the UK transport network: one is a composite in-situ concrete infill slab on precast prestressed concrete girders and the other is a composite bridge with steel girders and cast-in-situ reinforced concrete deck slab.
Data from the smart bridges has been collected, analysed and interpreted by structural engineers at Cambridge. Combining structural and data-driven engineering analysis brings new opportunities to smart infrastructure. Integrating these methods to take a data-centric approach delivers accurate and verified structural performance information to enable improved decision-making for asset managers.
Each bridge has the potential to generate up to 12GB of data per day. One goal of this project was to identify which of the data collected are important to retain and which could be discarded, developing automated techniques for culling unimportant data between train events and potentially from train events which provide no additional detail.
The combination of sophisticated data analytics techniques incorporated with advanced data visualisation and 3-D data projection models provided an opportunity to develop advanced digital twin bridge models.
Data collected from the monitoring systems was interpreted using integrated algorithms to provide additional insights into the site-specific conditions. For example, using the site monitoring data from the self-sensing bridge, the researchers developed a real-time train load monitoring system based on Bridge Weigh-In-Motion (B-WIM) technology. This predicts the individual axle weights of passing trains on the fly with above 95% accuracy. It is the first successful permanent train load monitoring system based on B-WIM technology. Having traffic loading information not only benefits operators for overload monitoring and control purposes, it also serves to generate an accurate digital representation of the structure. The traffic loading information can be imposed back into the calibrated physics-based model, within the digital twin system, to predict the stresses and deformations throughout the structure, including un-instrumented locations. The system can then predict the load rating factor of the entire structure and identify critical components for maintenance to secure better planning and efficiencies.
Process innovation
The digital infrastructure has been designed to be compatible with any cloud-computing platform, such as Microsoft Azure. This allows information generated from the digital twin system to be stored and processed securely. The cloud-compatible setup is coupled with a web-based front end, so that future clients can access and interact with live data reliably on the dashboard from their control room. Cloud computing creates resilience, enabling efficient collection, storage and processing of big data.
With the data collection and visualisation tools in place, artificial intelligence algorithms can extract useful information collected from multiple parts of the structure, feeding it back into the system to be further refined. Implementation of machine learning allows continuous simulation of various damage ‘what-if’ scenarios and conditions, generating a deterioration model of the bridge. Such a model supports bridge owners to proactively plan maintenance.
Meet the research team
Lead: Professor Campbell Middleton, Director, Laing O’Rourke Centre for Construction Engineering & Technology, University of Cambridge; Dr Jennifer Schooling OBE, Director, Centre for Smart Infrastructure and Construction, University of Cambridge
Team: Dr Farhad Huseynov, Senior Research Associate, Centre for Smart Infrastructure and Construction and Laing O’Rourke Centre for Construction Engineering & Technology; Paul Fidler, Computer Associate, Centre for Smart Infrastructure and Construction; Dr Didem Gürdür Broo; Dr Vladimir Vilde and Dr Miguel Angel Bravo-Haro, Research Associates, Centre for Smart Infrastructure and Construction
“The difference this research will make to industry is compelling. Digital twin technology will help the operators to better understand the structural behavior of bridges and reveal their remaining service life. Up until now, as an industry we haven't been able to do it. Now we have this opportunity to achieve something astounding.”
Dr Farhad Huseynov, Senior Research Associate, University of Cambridge