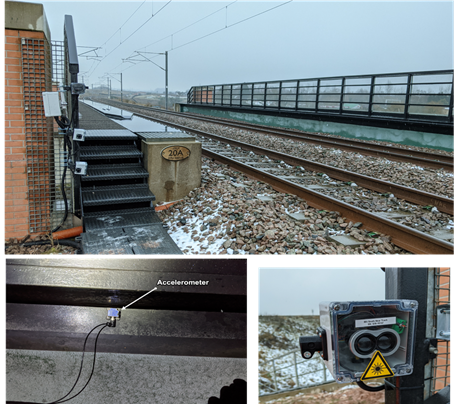
Submitted by Anonymous on Mon, 15/11/2021 - 11:34
Digital twins enable asset owners to use better information at the right time to make better decisions. Exploring the early stages of a digital twin journey – understanding the information need – are Staffordshire Bridges researcher Dr Farhad Huseynov and Head of Information Management Henry Fenby-Taylor.
Network Rail manages over 28,000 bridges, with many being more than 150 years old. The primary means of evaluating the condition of the bridges is through two assessment programmes; visual examination and Strength Capability Assessment. Every conceivable form of bridge construction is represented across Network Rail’s portfolio of assets, from simple stone slabs to large estuary crossings, such as the Forth Bridge. Managing a portfolio of this diversity with frequent and extensive assessments is a considerable challenge.
Condition monitoring
The current process for condition monitoring involves visual examination by engineers and takes place every year, along with a more detailed examination every six years. The visual inspection provides a qualitative outcome and does not directly predict the bridge strength; it is conducted to keep a detailed record of visible changes that may indicate deterioration. The load-carrying capacity of bridges is evaluated every five years through a Strength Capability Assessment, conducted in three levels of detail:
- Level 1 is the simplest, using safety assumptions known to be conservatively over-cautious (i.e. 1-dimensional structural idealisation).
- Level 2 involves refined analysis and better structural idealisation (i.e. grillage model). This level may also include the use of data on material strength based on recent material tests, etc.
- Level 3 is the most sophisticated level of assessment, requiring bridge-specific traffic loading information based on a statistical model of the known traffic.
Understanding the information and insights that asset owners require helps shape what data is needed and how frequently it should be collected – two essential factors in creating infrastructure that is genuinely smart. During the discussions with Network Rail, the research team found that Level 3 assessment is only used in exceptional circumstances. This is because there is no active live train load monitoring system on the network; hence there is no site-specific traffic loading information available for the majority of bridges. Instead, bridges failing Level 2 assessment are typically put under weight and/or speed restrictions, reducing their ability to contribute to the network. This means that there is potentially huge value in providing Level 3 assessment at key sites with greater frequency.
Digital twins for condition assessment
The Stafford Area Improvement Programme was setup to remove a bottleneck in the West Coast Main Line that resulted in high-speed trains being impeded by slower local passenger and goods trains. To increase network capacity and efficiency, a major upgrade of the line was undertaken, including the construction of 10 new bridges. Working with Atkins, Laing O’Rourke, Volker Rail and Network Rail, a research team including the Centre for Smart Infrastructure and Construction (CSIC), the Centre for Digital Built Britain (CDBB) and the Laing O’Rourke (LOR) Centre for Construction Engineering and Technology at the University of Cambridge is collaborating with Network Rail to find a digital twin solution for effective condition monitoring.
Two bridges in the scheme were built with a variety of different sensors to create a prototype that would enable the team to understand their condition, performance and utilisation. Both bridges were densely instrumented with fibre optic sensors during construction, enabling the creation of a digital twin of the bridges in use. The digital twin’s objective is to provide an effective condition monitoring tool for asset and route managers, using the sensor array to generate data and derive insights.
Identifying challenges and solutions
Meetings were held with key stakeholders including route managers and infrastructure engineers at Network Rail to learn the main challenges they face in maintaining their bridge stock, and to discover what information they would ideally like to obtain from an effective condition monitoring tool. The team liaised closely with the key stakeholders throughout to make sure that they were developing valuable insights.
Through discussions with Network Rail about the team’s work on the two instrumented bridges in the Staffordshire Bridges project the following fundamental issues and expected outcomes were identified:
- A better understanding of asset risks: How can these be predicted? What precursors can be measured and detected?
- A better understanding of individual asset behaviour
- Development of sensor technology with a lifespan and maintenance requirement congruent with the assets that they are monitoring
- How structural capability be calculated instantly on the receipt of new data from the field
- Development of a holistic system for the overall health monitoring and prognosis of structures assets
- Realistic traffic population data in the UK railway network. (Can this be predicted with sufficient accuracy for freight control and monitoring purposes?)
To address these issues, the team instrumented one of the bridges with the following additional sensors, which, combined, produce a rich dataset:
- Rangefinder sensors to obtain the axle locations.
- A humidity and temperature sensor to improve the accuracy of weight predictions against variations in ambient temperature.
- Accelerometers to calculate rotational restraints at the boundary conditions and therefore improve the accuracy of weight predictions.
- Cameras to categorise passing trains.
Data from these sensors feeds into a finite element model structural analysis digital twin that interprets the data and provides a range of insights about the performance of the bridge and the actual strain it has been put under.
Applying insights to other bridges
Significantly, information from the instrumented bridge sites is relevant to adjacent bridges on the same line. Having one bridge instrumented on a specific route would enable Level 3 assessment for other structures in their portfolio and those of other asset owners, including retaining walls, culverts, and other associated structures. Just as the new bridges relieved a service bottleneck, digital twins can resolve procedural and resource bottlenecks by enabling insights to be drawn about the condition of other assets that weren’t instrumented.
This is a valuable insight for those developing their own digital twins, because given that one bridge is instrumented it follows that where trains cannot have diverted course, then any other bridges along that same stretch of track will be undergoing the same strain from the same trains. This insight will enable teams implementing sensors to be able to efficiently implement a sensor network across their own assets.
One of the outcomes of the Staffordshire Bridges project is development towards a holistic approach for the overall health monitoring and prognosis of bridge stocks. Such changes improve workforce safety by reducing the requirement for costly site visits while maintaining a healthy bridge network.
You can read more from the Staffordshire Bridges project by visiting their research profile.
This research forms part of the Centre for Digital Built Britain’s (CDBB) work at the University of Cambridge. It was enabled by the Construction Innovation Hub, of which CDBB is a core partner, and funded by UK Research and Innovation (UKRI) through the Industrial Strategy Challenge Fund (ISCF).
To join the conversation with others who are on their own digital twin journeys, join the Digital Twin Hub.